Top 3 Approaches to Fraud Detection and Prevention Management During COVID-19 Pandemic
The outbreak of COVID-19 global pandemic has brought the world to a standstill. It has now become common to implement basic rules-based approaches to fraud detection and prevention management. The use of advanced technology such as artificial intelligence and machine learning is increased in the pandemic to detect frauds. An intelligent approach can impressively manage scale and deliver a high level of transparency.

The COVID-19 pandemic has brought the businesses and the world to a standstill. While communities are grouping together to support one another, not every person is working cohesively for the common good. Since, the novel coronavirus reached the pandemic level, cybercrime has spiked with Checkphish reporting a million of attacks in May 2020 rising from 400 attacks in February 2020.
Though technology has revolutionized the fraud detection departments, many approaches to fraud detection and prevention still fall flat when it comes to the high level of accuracy. But it is the organization and not the technology that should be held legally accountable for the decisions made. Thus, choosing a solution that delivers a high level of accuracy and accountability in fraud judgments is key. According to the Research Dive, the global fraud detection and prevention market is expected to observe additional growth in 2020. Originally, the global market was estimated to garner US$ 30.0 billion, but due to COVID-19 pandemic the market is now projected to reach up to US$ 31.5 billion by the end of 2020.
Here are some of the approaches to fraud detection and prevention management.
The Conventional Approach
It has now due to COVID-19 pandemic become common to adopt or implement basic rules-based approaches to fraud detection and prevention management. A logical program of sequential parameters is applied in basic rules-based systems as they use these parameters to detect instances of fraud and consequently perform automated actions.
The recent spike in advanced fraud cases means more sophisticated basic rules-based systems need to be adopted, as illustrated by the Association of Certified Fraud Examiners (ACFE), which estimated about 200% increase in the use of machine learning (ML) and artificial intelligence (AI) to detect fraud.
The Adaptive Approach
Machine learning has the capability to detect sophisticated frauds or fraudulent activities across huge or large volumes of data, ‘learning’ to become accustomed to new, unexpected threats, while considerably reducing false positives.
ML programs can be classified into two approaches
- Supervised: In supervised ML algorithms, one can present it with both fraudulent as well as non-fraudulent records. In COVID-19 pandemic, this ‘labelled’ data can help in achieving the level of accuracy to produce a data model, which will predict whether fraud is present when assessing unknown or new fraud cases. On the other hand, supervised learning is incapable of scaling on-demand to evolving threats as it requires significant computation time for training, clean data, and a data scientist’s team, to build, maintain, and interpret.
- Unsupervised: Unsupervised ML is useful for detecting fraudulent patterns where labelled data is not available. This model or approach will work off an untagged dataset, with unknown output values mapped with the input. It creates a function describing the structure of the data, and marks anything that doesn’t fit as an anomaly. This eventually saves time and enables the algorithm to mine at scale without any need for human input. However, a major drawback to unsupervised ML is its incapability to accurately explain its results, owing to the input data being unknown and unlabeled.
The Intelligent Approach
Intelligent automation or IA, via a visual model, copies the knowledge of the fraud experts and spreads over this knowledge to thousands of cases, while rapidly providing an audit trail for each decision. This includes its certainty level, data sources that were accessed, and every factor that goes into making each decision.
As compared to simple rules-based fraud detection tools, the modelling process in IA results in more reliable outcomes. An intelligent approach also handle uncertainties in the dataset. When the data is uncertain or missing, IA tries to find other data to help. If it is not able to find supporting data, IA is still able to make an inference, which will be unfilled with reduced certainty. Thus, unlike a fixed rules engine, IA doesn’t hit a dead end when data isn’t available.
In COVID-19 pandemic, an intelligent approach can impressively manage scale and deliver a high level of transparency. Moreover, COVID-19 won’t be the last widespread crisis the world will have to deal with. With the forthcoming risks of future pandemics, choosing the right fraud detection and prevention approach cannot be a matter of preference.
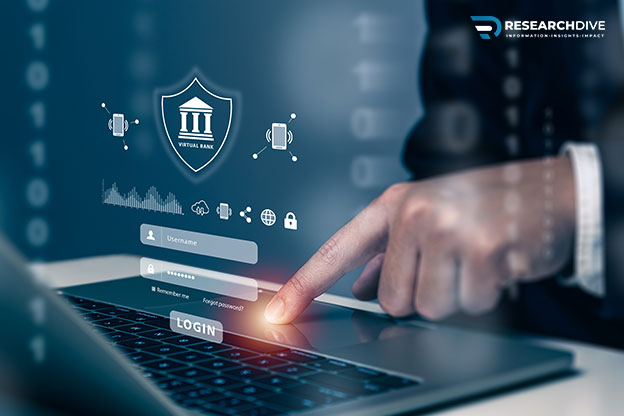
How is Cybersecurity Becoming a Vital Measure to Combat Emerging Threats in the Banking Sector Globally?
April 08, 2024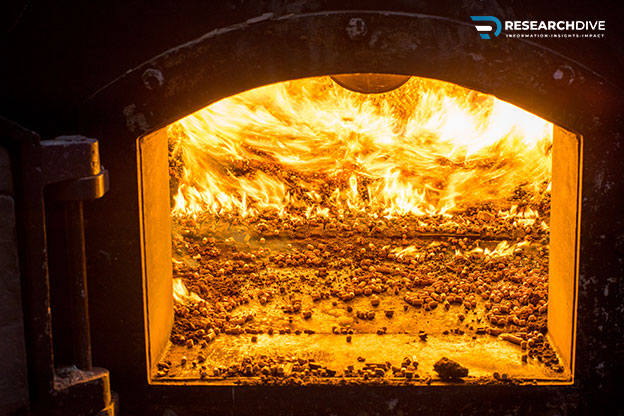
Wood Pellet Biomass Boilers: An Eco-Friendly Heating Solution
April 08, 2024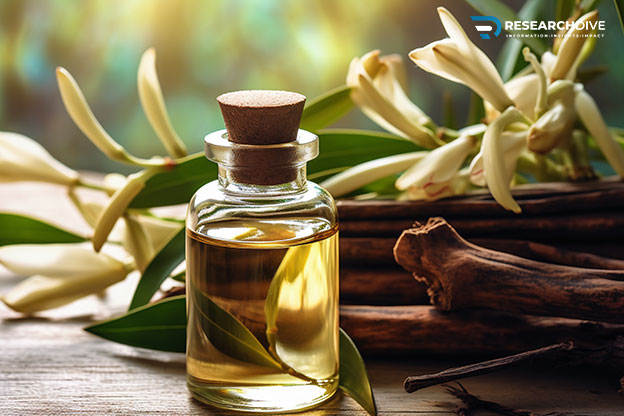
5 Ways Vanilla Oil Can Transform Your Life
April 08, 2024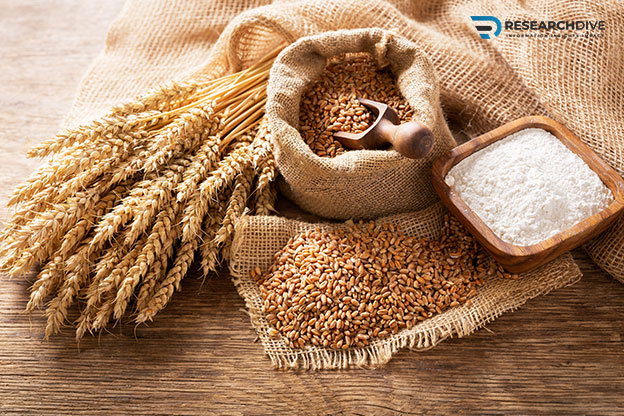
Discovering the Magic of Toasted Flour: Why & How to Use It
April 08, 2024