AI Accelerator Chip Makers in a Race to Develop the Most Energy-efficient AI Accelerator Chips
One of the trending topics in the field of AI accelerator chips is the increasing focus on energy efficiency and reducing the environmental impact of data centers. There is increasing competition among chip manufacturers to develop and market the most advanced and energy-efficient AI accelerator chips. With the increasing demand for artificial intelligence (AI) workloads, the market for AI accelerator chips has been expanding rapidly.

AI accelerator chips are specialized processors that are optimized for running artificial intelligence workloads, such as deep learning, computer vision, and natural language processing. One of the trending topics in the field of AI accelerator chips is the increasing focus on energy efficiency and reducing the environmental impact of data centers. As AI workloads become more computationally intensive and require more energy, there is a growing concern about the environmental footprint of data centers that support these workloads.
To address this issue, chipmakers are developing more energy-efficient AI accelerator chips that can provide high performance with lower power consumption, which is driving the growth of the AI accelerator chip market.
Some of the recent advancements in the AI accelerator chip industry:
- NVIDIA announced its latest AI accelerator chip, the NVIDIA A100 Tensor Core GPU, in May 2020. The A100 is designed for use in data centers and can deliver up to 20 times the performance of its predecessor. NVIDIA's A100 Tensor Core GPU uses a new architecture that delivers better energy efficiency than previous generations of GPUs.
- In July 2020, Intel launched its first AI-specific accelerator chip, the Intel Nervana NNP-T1000. The NNP-T1000 is designed for deep learning workloads and features a specialized tensor processor, which is a type of processor that is optimized for matrix operations that are commonly used in neural networks. The NNP-T1000 is built on a new architecture that is optimized for deep learning workloads, with a focus on high performance and energy efficiency. Overall, the Intel Nervana NNP-T1000 is an important development in the field of AI accelerator chips, as it represents a significant step forward in the design and optimization of hardware for deep learning workloads. Its specialized tensor processor and high-bandwidth memory make it an ideal chip for large-scale deep learning workloads, while its programmability makes it highly adaptable and versatile.
- In May 2021, Google announced the release of its latest AI accelerator chip, the Tensor Processing Unit (TPU) v4. This chip is designed to power large-scale artificial intelligence workloads, such as deep learning, natural language processing, and computer vision. The TPU v4 is a significant improvement over its predecessor, the TPU v3, with the ability to deliver up to 4 petaflops of computing power. This is achieved through a combination of improvements in chip design, manufacturing, and packaging, which allow for higher performance and energy-efficient versatile chip that can be used to accelerate a wide range of deep learning workloads in data centers.
In addition to architecture, chipmakers are also exploring new materials and manufacturing techniques that can improve energy efficiency. For example, some chipmakers are using new semiconductor materials, such as gallium nitride (GaN), which can reduce power consumption and increase performance. Others are exploring 3D packaging technology, which can reduce the distance that electrical signals must travel between components, thus reducing power consumption.
Overall, the focus on energy efficiency in the development of AI accelerator chips is an important trend, as it can help to reduce the environmental impact of data centers and make AI more sustainable in the long term.
The Way Ahead for AI Accelerator Chip Market
With the increasing demand for artificial intelligence (AI) workloads, the market for AI accelerator chips has been expanding rapidly. As per a report by Research Dive, the global AI accelerator chip market is expected to grow with a CAGR of 39.3% in the 2022-2031 timeframe, by surpassing $332,142.7 million by 2031.
The COVID-19 pandemic has also played a role in the growth of the AI accelerator chip market, as it has accelerated the adoption of AI and other digital technologies in various industries. For example, AI has been used in medical research to help develop treatments and vaccines for COVID-19.
Overall, the AI accelerator chip market is expected to continue to grow in the coming years, driven by the increasing demand for AI applications and the ongoing development of new and more advanced chips.
The Bottom Line
There is increasing competition among chip manufacturers to develop and market the most advanced and energy-efficient AI accelerator chips. Market players are investing heavily in research and development to create specialized processors that are optimized for running AI workloads. This is leading to a constant stream of new products and innovations in the market. Overall, the race to develop the most energy-efficient AI accelerator chips is driving significant innovation and competition in the industry. This competition is resulting in the development of new technologies and solutions that are making AI more accessible and efficient.
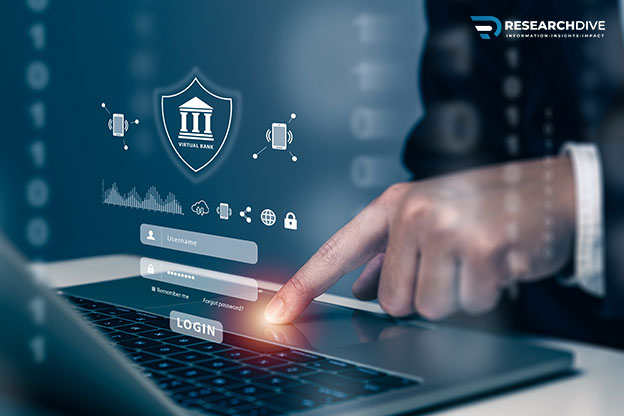
How is Cybersecurity Becoming a Vital Measure to Combat Emerging Threats in the Banking Sector Globally?
April 08, 2024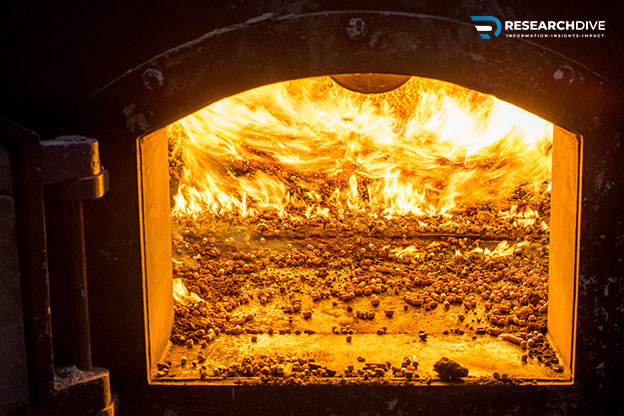
Wood Pellet Biomass Boilers: An Eco-Friendly Heating Solution
April 08, 2024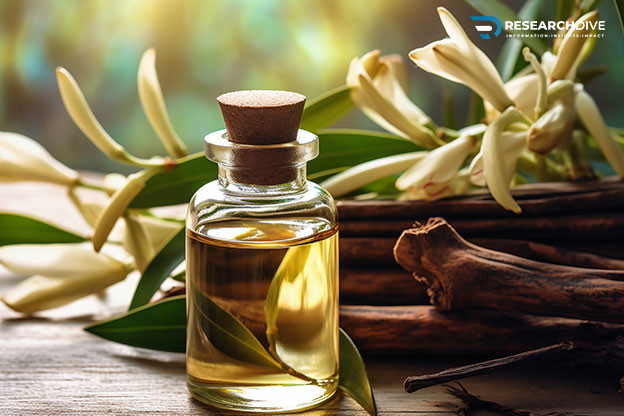
5 Ways Vanilla Oil Can Transform Your Life
April 08, 2024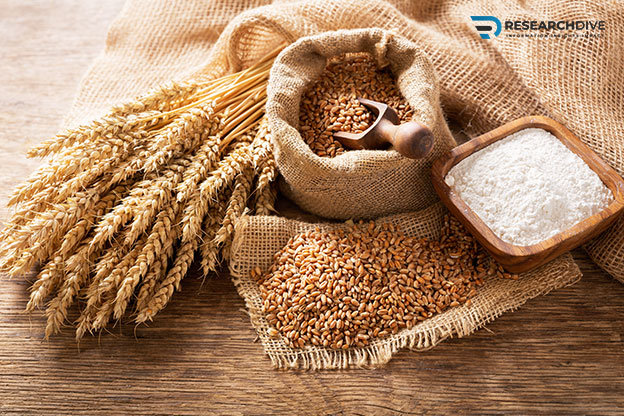
Discovering the Magic of Toasted Flour: Why & How to Use It
April 08, 2024